FIX YOUR FUNNEL PLAYBOOK
Decreasing rejection rate with enrichment, targeting and alignment
Struggling with your lead rejection rate from sales?
That was the problem Steve Armenti had whilst working at Google Chrome Enterprise. Here's how he used a combination of data enrichment, targeting and alignment to slash that rejection rate from 90% to 40%.
Industry and company size
-
10k+ employees
-
SaaS
Funnel stage
-
MQL > SQO
Playbook impact
-
90% > 40% rejection rate
PLAYBOOK HOST
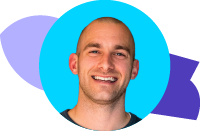
Steve Armenti
VP of Revenue Marketing @Digital Ocean
RECOMMENDED FOR
Let's jump in 👇🏻
💡 What was the problem?
Steve and his team at Google Chrome Enterprise were tasked with generating MQLs to send over to their sales team to follow up on. At the time, the team had ramped up their lead generation - buying leads from multiple vendors.
Only to find that a huge percentage of them - around 87% - would be rejected, not converting to SQLs. This was abnormally high for the business, so it was quickly highlighted in a monthly pipeline review meeting.
💡 Auditing the quality of MQLs and scoring
Steve and the rest of the marketing team initially felt that this couldn’t have been a problem with MQL quality.
They were sending MQLs through that matched predetermined criteria, so it must have been an issue with the sales team training.
To validate this, they reviewed the sales team’s processes. And yes, they did find small areas where improvements could be made in training, e.g. using the correct templates and avoiding typos in emails.
But for the most part, they couldn’t explain the rejection rate. Which meant they had to consider that this was an issue of MQL quality.
Steve and his team manually went through 1,500 MQLs. One of the main evaluation criteria was ‘would I call this MQL if I were a sales rep?’, if the answer was no, adding the reasons why, into a large spreadsheet, e.g. wrong job title, contact data not complete, etc.
Steve said:
“I was humbled, in reality, the vast majority were low quality and we had been sending misfit leads through to the sales team.”
In this case, the lead scoring mechanism marketing had been using to validate the leads coming from vendors was causing a lot of confusion. Because the system was scoring accounts as a good fit, when ultimately, they’d never have closed for a number of reasons.
Steve said:
“If you’re pumping a bunch of bad data into lead scoring, e.g. here’s someone who's a VP so lead scoring says ‘yes this person is great for us’, but in reality we don’t have complete contact data for this person - then how good is that lead?”
💡 Redefining ICP, systems and using enrichment
There were a number of elements to the solution that Steve and his team implemented:
1) Redefine the ICP, which could then be translated back to the vendors supplying the leads.
Steve said:
“Our vendor had been sending over a bunch of bad leads, but it wasn’t their fault because we gave them a really broad description of the ICP.”
This meant going back to the drawing board and redefining exactly who they wanted to bring in.
For example, the job titles they wanted to get. Which was mid-level decision makers, IT managers for example. Not CTOs or anyone more senior, as they wouldn’t be involved in the purchasing of Chromebooks.
2) Setting up proper governance over what leads made it through.
In other words, setting up rules in systems meaning that only leads with full contact information (not just HQ reception numbers!) would be considered an MQL.
3) Alongside this, the team focused on enriching the leads that they received to ensure the information they had was the most up to date as possible.
4) Finally, the last piece of the puzzle was to tighten up the rejection reasons recorded from the sales team.
Prior to the new adjustments being made, the majority of rejections were recorded as ‘couldn’t connect’ because the reps couldn’t reach the prospect.
On the surface, that meant they called the rep 12 times but they never answered the phone. But when this data was investigated, it was revealed that the rep had called an 1800 number - so of course they hadn’t been able to reach a decision maker.
Another common rejection reason that was often used was ‘not qualified’ but this didn’t offer any real insights as to why they weren’t qualified. For example, was it the prospect saying they didn’t have the budget?
Instead, reps were trained on how to properly log reasons why they couldn’t get hold of prospects and why they were rejecting prospects so this data could be better used to monitor performance.
💡 What were the results?
Steve and the team still used rejection rate as their north star metric. They managed to get this down from nearing 90% down to below 40% after around 8-9 months of implementing these changes.
Steve said:
“Because we had made all of these tweaks to make sure the sales team were getting the best data possible, we were confident that when the sales team marked a lead as ‘could not connect’, that this was now for a legitimate reason.”
Naturally as the rejection rate went down, the sales qualification rate went up.
Steve added:
“At the start of the year, our SQL metric was on track - but then we invested more in lead gen and our SQLs dropped in Q2.”
“But by the time I left Google Chrome Enterprise we hit our SQL number for the year. But it was only because of that intervention that we managed to hit our goal.”